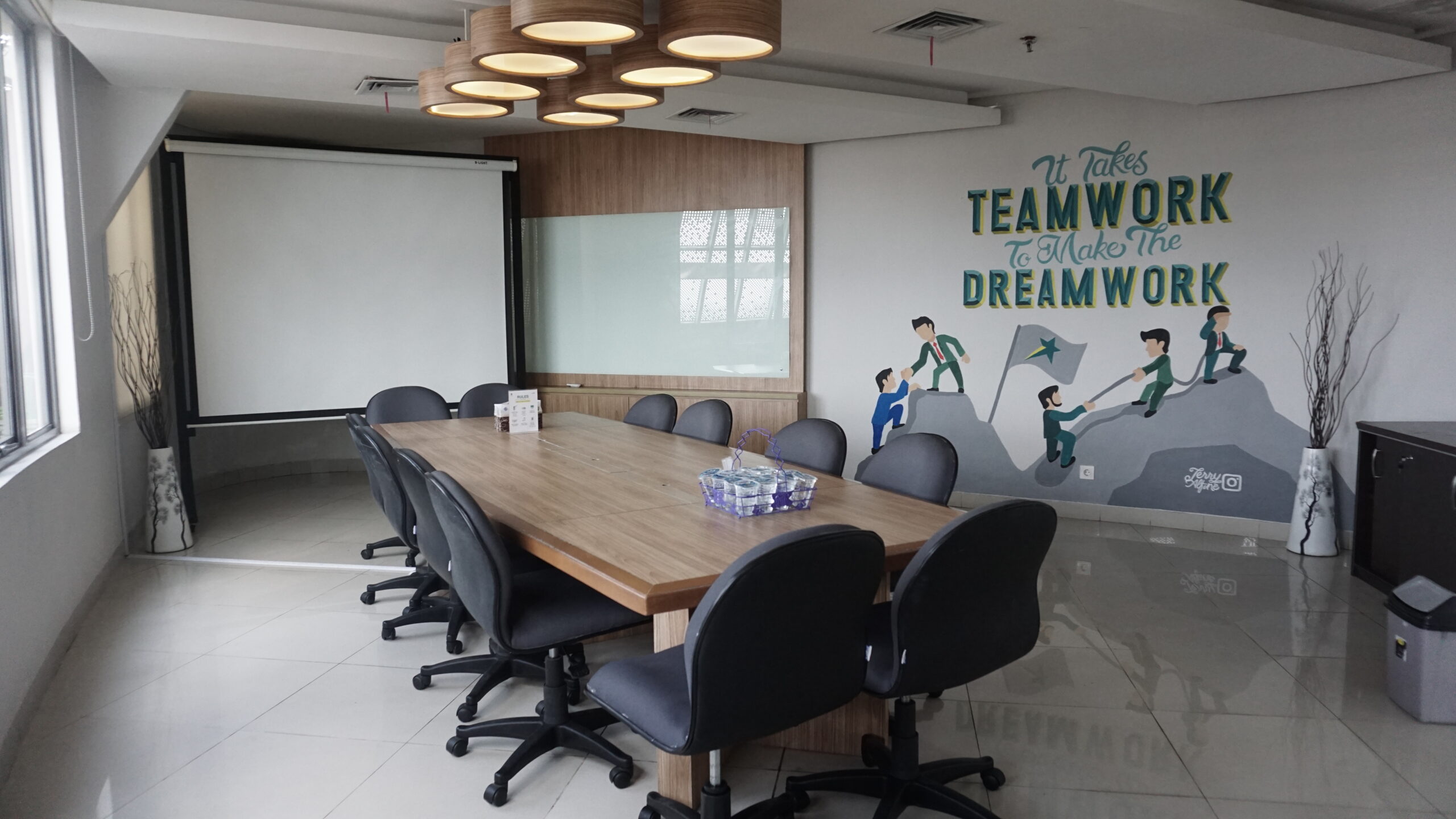
Want To Be Productive? Come Try Working From The Most Cozy Coworking Space in Tangerang
March 7, 2022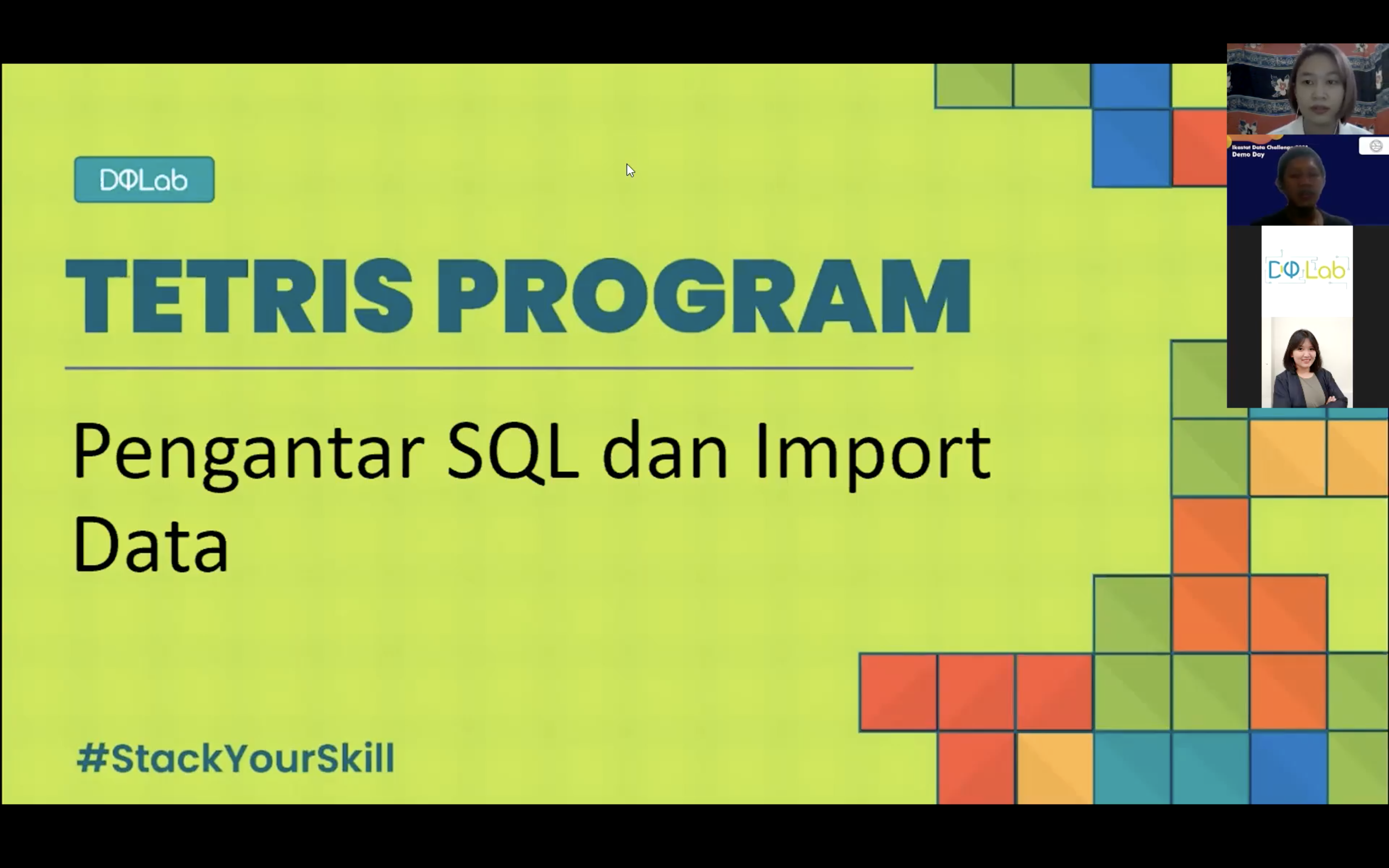
Learn SQL, An Essential Skill for Data Practitioners
March 7, 2022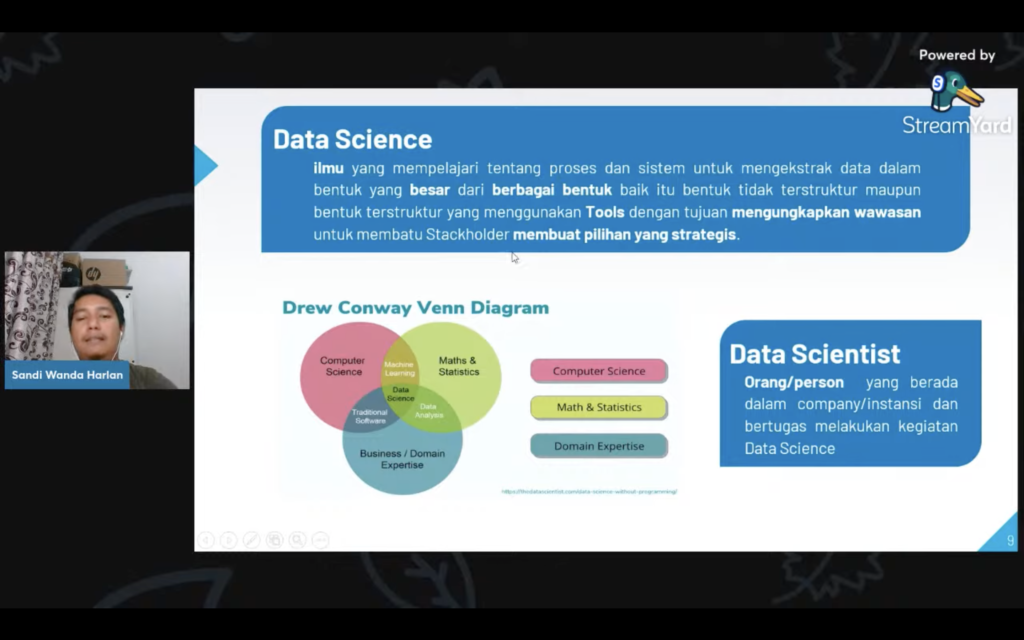
Sandi Wanda Harlan selaku Region Credit Analyst di Fifgroup member of Astra pembicara di acara Opening Live Session Bootcamp DQLab. (dok. DQLab)
TANGERANG – Opening the Live Session Bootcamp, DQLab introduced the world of data science through a mini Bootcamp held virtually on Monday (13/12/21). The Bootcamp invited keynote speaker Sandi Wanda Harlan, a Region Credit Analyst at the Federal International Finance (FIFGROUP), member of Astra.
In this event, Sandi’s main focus of discussion was the difference between data science and data scientist, data analyst, and data engineer. He also explained why we should learn data science and how to learn it effectively.
Data is a collection of information obtained through observation or searching from specific sources. Initially, the science of studying data is done with statistics. But, as time goes, there needs more development and growth to solve problems. Eventually, data science emerged as an interdisciplinary science that includes statistics, programming, and science related to certain fields. Interdisciplinary here means that it can be related to other sciences related to data.
Data science studies the processes and systems that process big data using tools to find insights to help stakeholders make choices. The person in charge of processing data using data science is called a Data Scientist.
“When learning data science, it’s crucial to master computer science, maths, and statistics,” Sandi emphasised.
There are several processes in data science, starting from collecting data, processing data, cleaning data, analyzing exploratory data to making models with algorithms and communicating data through data visualization. To provide an overview of the data science lifecycle, Sandi provided examples of the application of data science in several fields.
“For example, system recommendations or determining rates and discounts in the e-commerce industry. Consumer segmentation or market basket analysis in the retail industry. Detecting credit or analyzing creditworthiness. Detecting images and videos or sentiment analysis in social science. And lastly, detecting diseases or insurance approval in the medical industry,” Sandi explained.
According to Sandi, a data scientist is needed to this day because it is still a very promising occupation. They learn about the processes and systems to process data to assist in decision-making, making them essential in business strategies and being able to predict trends in the future. Other professions like data engineering and data analyst are also essential in the lifecycle of data science.
Also read Learning Data Science: Tips To Become a Data Practitioner Targeted by Companies
Data Engineers is in charge of developing and designing the data management architecture and maintaining the company’s data infrastructure. While Data Analysts is responsible for processing data, drawing conclusions and visualizing data, to find insights from a business perspective.
Sandi continued explaining the roles of data scientists, data engineers and data analysts when working together in the data science lifecycle.
In the data science lifecycle, data engineers collect data, process data, clean data, create data products, and return them to reality. Meanwhile, data analysts clean data, analyse exploratory data, and communicate and visualise data. And lastly, data scientists processes data, cleans data, analyzes exploratory data, makes data models, also communicates and visualizes data.
Sandi also mentioned several tools used by the three data practitioners. Data engineers must be proficient in R, Python, SQL, BigQuery and Pentaho. Data analysts must be able to use Spreadsheets, Tableau, Power BI, SQL and Google Analytics. Tools commonly used by data scientists are R, Python, SQL, Machine Learning and Java. SQL becomes very important for data practitioners in processing data.
Learning data science takes some hard skills such as statistics, programming, and related knowledge. While the soft skills needed are curiosity, can provide arguments, and storytelling data.
“For beginners, it’s okay to learn the basics of statistics. Because the most widely used for data exploration are basic ones such as the average value, maximum value, minimum value,” said Sandi.
Related knowledge is also important. What this means is that, for example, when dealing with retail data, you must be knowledgeable about the retail sector. This applies to other fields.
For beginners, especially non-IT beginners, Sandi advised learning the basics of data science. This can be done by joining groups in social media, courses and webinars, daring to participate in competitions to create portfolios, and being consistent when learning and practising.
Implement Sandi’s data science learning tips and tricks by taking courses and being brave to practice and be consistent in learning with DQLab.
by Lathifa Lisa | DQLab
Kuliah di Jakarta untuk jurusan program studi Informatika| Sistem Informasi | Teknik Komputer | Teknik Elektro | Teknik Fisika | Akuntansi | Manajemen| Komunikasi Strategis | Jurnalistik | Desain Komunikasi Visual | Film dan Animasi | Arsitektur | D3 Perhotelan | International Program, di Universitas Multimedia Nusantara. www.umn.ac.id