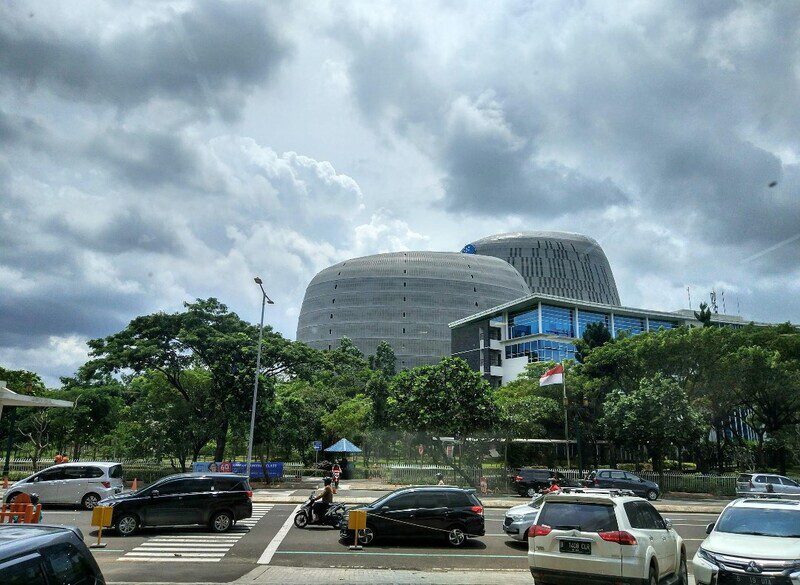
Often Considered the Same, Here’s The Difference Between Academy VS University
August 16, 2022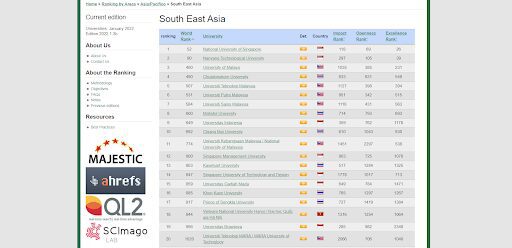
The Importance of University Rankings, Here’s a Complete Explanation of Webometrics Ranking
August 16, 2022Tangerang – R is one of the programming languages used to process data and graphics. R is considered quite popular and is often used by data practitioners because it has many features, is easy to learn, is faster, is free, and open-sourced. Encouraging the use of R as a foundation when learning data science, DQLab presented a virtual live session discussing “R Fundamentals for Data Science.” This live session was held on Thursday, July 21, 2022, with Shella Pandiangan, a Data Scientist at United Tractor, as a speaker. Shella introduced R in data science.
The first discussion is about the introduction of data science. According to Shella, Data Science consists of two words: data and science. Data is closely related to things around us, for example, email messages, while science is the science/knowledge. It can be concluded that data science is the science of processing data. Recently, data science has become very popular, especially in Indonesia. Many Indonesians have mastered data processing skills.
People who master data processing can be called a data team. The data team consists of a Data Scientist, Data Engineering, and Data Analyst. Data Analysts usually process data based on the problems and generate insights from the problems. A Data Analyst requires skills in statistics, communication, and business knowledge. A Data Engineer is someone responsible for tidying and cleaning data. The skills needed by a Data Engineer are programming, mathematics, and big data. A Data Scientist is in charge of modeling. Data Scientist is expected to master statistics, mathematics, programming, and communication skills. Before the Data Scientist and Data Analyst work, the Data Engineer must first process the data.
“Generally when a job vacancy appears, they usually look for a Data Scientist who understands R, Python or SQL,” Shella said.
Data science is a multidisciplinary discipline of several sciences. To become a data practitioner, you need data science that covers many areas, such as machine learning, big data, mathematics, statistics, traditional research, subject matter expertise, and programming. So, in data science, you will not only learn mathematics and statistics but will also be combined with business knowledge or traditional research. These sciences will help a data practitioner answer existing problems.
Understanding data science can be started by understanding the problem, analyzing the problem in business, making a business strategy, doing domain knowledge, and finally, the domain knowledge is communicated and presented to the user.
“Data science is a science. People who use data knowledge are not only Data Scientists, but also Data Analysts, Marketing Analysts, and Marketing Intelligence. So, if we study data science, we don’t have to be a Data Scientist,” Shella said.
A good Data Scientist must have inquisitive (curiosity), is knowledgeable, understand scientific method, coding, is product-oriented, and domain knowledge. Knowledgeable means understanding machine learning, statistics, and probability because when you enter the world of data, you need these knowledge. Scientific method means you have to understand how to make hypotheses and how to test hypotheses. Coding means a Data Scientist must understand coding, but it doesn’t have to be detailed but understand programmings like R or Python. Product-Oriented means that they must be able to build data products and data visualizations so that the data is easy to understand.
Back again to the discussion of data science, there have been many uses of data science in various fields, such as travel, marketing, healthcare, social media, sales, automation and credit, and insurance. Data science can be used for dynamic pricing and predicting delayed flights in the travel business. In marketing, data science can be used to predict customers, cross-selling, and upselling. In healthcare, data science can be used to predict diseases. In social media, data science is used for digital marketing and sentiment analysis. In sales, data science is used for demand forecasting and discount offerings. In automation, data science can be used to create machine learning models for self-driving cars, pilotless aircraft, and drones. Finally, in credit and insurance, data science can be used for claims prediction.
“With data science we can retrieve important information, because data-based information can increase company value. Data science also helps us to get to know our customers better,” Shella said.
Moving on to the discussion of programming for data science. In this discussion, Shella focuses on R because R is a programming language designed for data science (analyzing data). In addition, R also has many functions or libraries, can connect to various databases, is suitable for data visualization and reporting, and is easy to create machine learning models.
“Whatever the tools, the knowledge remains the same,” Shella said.
Another advantage when using R is that many people use R. Automatically, many R communities are available to help solve problems. R is also friendly for prospective data practitioners new to the world of data. And many large companies have used R as a tool to help solve company problems. This opens an opportunity for novice data practitioners to learn R as one of the fundamentals for a career in data science. R for Data Science can be studied at DQLab. In addition to learning together, in DQLab, beginners can discover and join data science communities.
Kuliah di Jakarta untuk jurusan program studi Informatika| Sistem Informasi | Teknik Komputer | Teknik Elektro | Teknik Fisika | Akuntansi | Manajemen| Komunikasi Strategis | Jurnalistik | Desain Komunikasi Visual | Film dan Animasi | Arsitektur | D3 Perhotelan , di Universitas Multimedia Nusantara. www.umn.ac.id